图书介绍
INTRODUCTORY ECONOMETRICS FOR FINANCE:SECOND EDITION【2025|PDF|Epub|mobi|kindle电子书版本百度云盘下载】
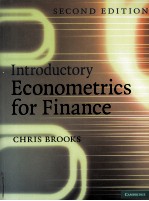
- 著
- 出版社:
- ISBN:9780521694681
- 出版时间:2008
- 标注页数:648页
- 文件大小:170MB
- 文件页数:671页
- 主题词:
PDF下载
下载说明
INTRODUCTORY ECONOMETRICS FOR FINANCE:SECOND EDITIONPDF格式电子书版下载
下载的文件为RAR压缩包。需要使用解压软件进行解压得到PDF格式图书。建议使用BT下载工具Free Download Manager进行下载,简称FDM(免费,没有广告,支持多平台)。本站资源全部打包为BT种子。所以需要使用专业的BT下载软件进行下载。如BitComet qBittorrent uTorrent等BT下载工具。迅雷目前由于本站不是热门资源。不推荐使用!后期资源热门了。安装了迅雷也可以迅雷进行下载!
(文件页数 要大于 标注页数,上中下等多册电子书除外)
注意:本站所有压缩包均有解压码: 点击下载压缩包解压工具
图书目录
1 Introduction1
1.1 What is econometrics?1
1.2 Is financial econometrics different from ‘economic econometrics?2
1.3 Types of data3
1.4 Returns in financial modelling7
1.5 Steps involved in formulating an econometric model9
1.6 Points to consider when reading articles in empirical finance10
1.7 Econometric packages for modelling financial data11
1.8 Outline of the remainder of this book22
1.9 Further reading25
Appendix:Econometric software package suppliers26
2 A brief overview of the classical linear regression model27
2.1 What is a regression model?27
2.2 Regression versus correlation28
2.3 Simple regression28
2.4 Some further terminology37
2.5 Simple linear regression in EViews-estimation of an optimal hedge ratio40
2.6 The assumptions underlying the classical linear regression model43
2.7 Properties of the OLS estimator44
2.8 Precision and standard errors46
2.9 An introduction to statistical inference51
2.10 A special type of hypothesis test:the t-ratio65
2.11 An example of the use of a simple t-test to test a theory in finance:can US mutual funds beat the market?67
2.12 Can UK unit trust managers beat the market?69
2.13 The overreaction hypothesis and the UK stock market71
2.14 The exact significance level74
2.15 Hypothesis testing in EViews-example 1:hedging revisited75
2.16 Estimation and hypothesis testing in EViews-example 2:the CAPM77
Appendix:Mathematical derivations of CLRM results81
3 Further development and analysis of the classical linear regression model88
3.1 Generalising the simple model to multiple linear regression88
3.2 The constant term89
3.3 How are the parameters(the elements of the β vector)calculated in the generalised case?91
3.4 Testing multiple hypotheses:the F-test93
3.5 Sample EViews output for multiple hypothesis tests99
3.6 Multiple regression in EViews using an APT-style model99
3.7 Data mining and the true size of the test105
3.8 Goodness of fit statistics106
3.9 Hedonic pricing models112
3.10 Tests of non-nested hypotheses115
Appendix 3.1:Mathematical derivations of CLRM results117
Appendix 3.2:A brief introduction to factor models and principal components analysis120
4 Classical linear regression model assumptions and diagnostic tests129
4.1 Introduction129
4.2 Statistical distributions for diagnostic tests130
4.3 Assumption 1:E(ut)=0131
4.4 Assumption 2:var(ut)=σ2<∞132
4.5 Assumption 3:cov(ui,uj)=0 for i≠j139
4.6 Assumption 4:the xt are non-stochastic160
4.7 Assumption 5:the disturbances are normally distributed161
4.8 Multicollinearity170
4.9 Adopting the wrong functional form174
4.10 Omission of an important variable178
4.11 Inclusion of an irrelevant variable179
4.12 Parameter stability tests180
4.13 A strategy for constructing econometric models and a discussion of model-building philosophies191
4.14 Determinants of sovereign credit ratings194
5 Univariate time series modelling and forecasting206
5.1 Introduction206
5.2 Some notation and concepts207
5.3 Moving average processes211
5.4 Autoregressive processes215
5.5 The partial autocorrelation function222
5.6 ARMA processes223
5.7 Building ARMA models:the Box-Jenlkins approach230
5.8 Constructing ARMA models in EViews234
5.9 Examples of time series modelling in finance239
5.10 Exponential smoothing241
5.11 Forecasting in econometrics243
5.12 Forecasting using ARMA models in EViews256
5.13 Estimating exponential smoothing models using EViews258
6 Multivariate models265
6.1 Motivations265
6.2 Simultaneous equations bias268
6.3 So how can simultaneous equations models be validly estimated?269
6.4 Can the original coefficients be retrieved from the πs?269
6.5 Simultaneous equations in finance272
6.6 A definition of exogeneity273
6.7 Triangular systems275
6.8 Estimation procedures for simultaneous equations systems276
6.9 An application of a simultaneous equations approach to modelling bid-ask spreads and trading activity279
6.10 Simultaneous equations modelling using EViews285
6.11 Vector autoregressive models290
6.12 Does the VAR include contemporaneous terms?295
6.13 Block significance and causality tests297
6.14 VARs with exogenous variables298
6.15 Impulse responses and variance decompositions298
6.16 VAR model example:the interaction between property returns and the macroeconomy302
6.17 VAR estimation in EViews308
7 Modelling long-run relationships in finance318
7.1 Stationarity and unit root testing318
7.2 Testing for unit roots in EViews331
7.3 Cointegration335
7.4 Equilibrium correction or error correction models337
7.5 Testing for cointegration in regression:a residuals-based approach339
7.6 Methods of parameter estimation in cointegrated systems341
7.7 Lead-lag and long-term relationships between spot and futures markets343
7.8 Testing for and estimating cointegrating systems using the Johansen technique based on VARs350
7.9 Purchasing power parity355
7.10 Cointegration between international bond markets357
7.11 Testing the expectations hypothesis of the term structure of interest rates362
7.12 Testing for cointegration and modelling cointegrated systems using EViews365
8 Modelling volatility and correlation379
8.1 Motivations:an excursion into non-linearity land379
8.2 Models for volatility383
8.3 Historical volatility383
8.4 Implied volatility models384
8.5 Exponentially weighted moving average models384
8.6 Autoregressive volatility models385
8.7 Autoregressive conditionally heteroscedastic(ARCH)models386
8.8 Generalised ARCH(GARCH)models392
8.9 Estimation of ARCH/GARCH models394
8.10 Extensions to the basic GARCH model404
8.11 Asymmetric GARCH models404
8.12 The GJR model405
8.13 The EGARCH model406
8.14 GJR and EGARCH in EViews406
8.15 Tests for asymmetries in volatility408
8.16 GARCH-in-mean409
8.17 Uses of GARCH-type models including volatility forecasting411
8.18 Testing non-linear restrictions or testing hypotheses about non-linear models417
8.19 Volatility forecasting:some examples and results from the literature420
8.20 Stochastic volatility models revisited427
8.21 Forecasting covariances and correlations428
8.22 Covariance modelling and forecasting in finance:some examples429
8.23 Historical covariance and correlation431
8.24 Implied covariance models431
8.25 Exponentially weighted moving average model for covariances432
8.26 Multivariate GARCH models432
8.27 A multivariate GARCH model for the CAPM with time-varying covariances436
8.28 Estimating a time-varying hedge ratio for FTSE stock index returns437
8.29 Estimating multivariate GARCH models using EViews441
Appendix:Parameter estimation using maximum likelihood444
9 Switching models451
9.1 Motivations451
9.2 Seasonalities in financial markets:introduction and literature review454
9.3 Modelling seasonality in financial data455
9.4 Estimating simple piecewise linear functions462
9.5 Marlkov switching models464
9.6 A Markov switching model for the real exchange rate466
9.7 A Marlkov switching model for the gilt-equity yield ratio469
9.8 Threshold autoregressive models473
9.9 Estimation of threshold autoregressive models474
9.10 Specification tests in the context of Markov switching and threshold autoregressive models:a cautionary note476
9.11 A SETAR model for the French franc-German mark exchange rate477
9.12 Threshold models and the dynamics of the FTSE 100 index and index futures markets480
9.13 A note on regime switching models and forecasting accuracy484
10 Panel data487
10.1 Introduction-what are panel techniques and why are they used?487
10.2 What panel techniques are available?489
10.3 The fixed effects model490
10.4 Time-fixed effects models493
10.5 Investigating banking competition using a fixed effects model494
10.6 The random effects model498
10.7 Panel data application to credit stability of banks in Central and Eastern Europe499
10.8 Panel data with EViews502
10.9 Further reading509
11 Limited dependent variable models511
11.1 Introduction and motivation511
11.2 The linear probability model512
11.3 The logit model514
11.4 Using a logit to test the pecking order hypothesis515
11.5 The probit model517
11.6 Choosing between the logit and probit models518
11.7 Estimation of limited dependent variable models518
11.8 Goodness of fit measures for linear dependent variable models519
11.9 Multinomial linear dependent variables521
11.10 The pecking order hypothesis revisited-the choice between financing methods525
11.11 Ordered response linear dependent variables models527
11.12 Are unsolicited credit ratings biased downwards?An ordered probit analysis528
11.13 Censored and truncated dependent variables533
11.14 Limited dependent variable models in EViews537
Appendix:The maximum likelihood estimator for logit and probit models544
12 Simulation methods546
12.1 Motivations546
12.2 Monte Carlo simulations547
12.3 Variance reduction techniques549
12.4 Bootstrapping553
12.5 Random number generation557
12.6 Disadvantages of the simulation approach to econometric or financial problem solving558
12.7 An example of Monte Carlo simulation in econometrics:deriving a set of critical values for a Dickey-Fuller test559
12.8 An example of how to simulate the price of a financial option565
12.9 An example of bootstrapping to calculate capital risk requirements571
13 Conducting empirical research or doing a project or dissertation in finance585
13.1 What is an empirical research project and what is it for?585
13.2 Selecting the topic586
13.3 Sponsored or independent research?590
13.4 The research proposal590
13.5 Working papers and literature on the internet591
13.6 Getting the data591
13.7 Choice of computer software593
13.8 How might the finished project look?593
13.9 Presentational issues597
14 Recent and future developments in the modelling of financial time series598
14.1 Summary of the book598
14.2 What was not covered in the book598
14.3 Financial econometrics:the future?602
14.4 The final word606
Appendix 1 A review of some fundamental mathematical and statistical concepts607
A1 Introduction607
A2 Characteristics of probability distributions607
A3 Properties of logarithms608
A4 Differential calculus609
A5 Matrices611
A6 The eigenvalues of a matrix614
Appendix 2 Tables of statistical distributions616
Appendix 3 Sources of data used in this book628
References629
Index641
热门推荐
- 2566874.html
- 866328.html
- 3768689.html
- 824083.html
- 3217751.html
- 357240.html
- 915441.html
- 1228371.html
- 3774456.html
- 668053.html
- http://www.ickdjs.cc/book_1349323.html
- http://www.ickdjs.cc/book_2479794.html
- http://www.ickdjs.cc/book_679403.html
- http://www.ickdjs.cc/book_742080.html
- http://www.ickdjs.cc/book_3863617.html
- http://www.ickdjs.cc/book_2383771.html
- http://www.ickdjs.cc/book_2378883.html
- http://www.ickdjs.cc/book_1117758.html
- http://www.ickdjs.cc/book_3718115.html
- http://www.ickdjs.cc/book_1037189.html